Measuring the Intention to Adoption of Environmental Sustainability in FMCG Enterprises using the Technology Acceptance Model (TAM)
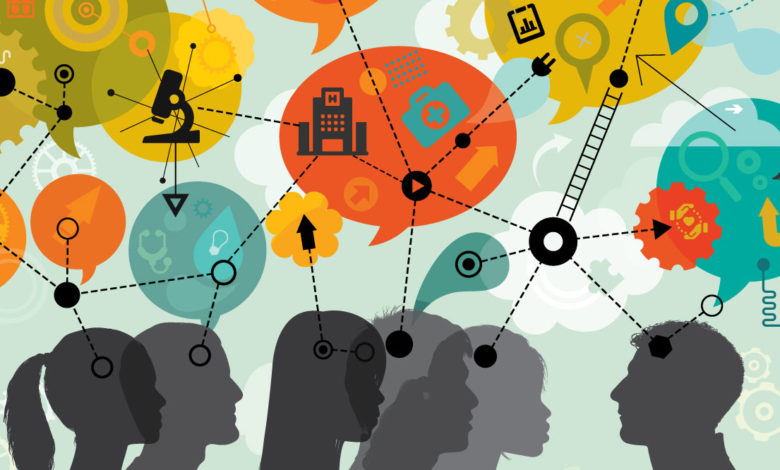
Prepared by the researche
- Dr. M. Salem N. Ismael – Doctorate Degree of Business Administration – “INTERNATIONAL BUSINESS SCHOOL OF SCANDNIVIA” (IPSS)- Denmark
- Dr. Ashraf H. Shaarawy – Doctorate Degree of Business Administration – “Ecole Supérieure Libre des Sciences Commerciales Appliquées” (ESLSCA) University- Egypt
- Dr. Mary Louis Rizk – Doctorate Degree of Business Administration – BLOOM BUSIENSS SCHOOL(BBS) CEO
Democratic Arabic Center
Journal of Afro-Asian Studies : Twenty-two Issue – August 2024
A Periodical International Journal published by the “Democratic Arab Center” Germany – Berlin
:To download the pdf version of the research papers, please visit the following link
Abstract
Using the Technology Acceptance Model (TAM) as a theoretical framework, this research explores how Fast-Moving Consumer Goods (FMCG) firms intend to implement environmental sustainability practices. A strong positive association between perceived usefulness and the behavioral intention to adopt environmental sustainability practices is established by research using correlation analysis, linear regression analysis, and multiple regression. Perceived ease of use also has a favorable effect on the behavioral intention to implement sustainability practices, according to the data. The results provide a detailed understanding of the elements influencing the adoption of sustainability in the fast-moving consumer goods (FMCG) industry, which is useful in both the academic and practical spheres. The research finishes with practical suggestions for moving forward, such as new ways of communicating and training employees, involving stakeholders, creating incentive programs, and incorporating sustainability into company culture.
These models have been widely applied and extended in various studies to explore technology acceptance across different sectors and applications. For instance, the application of these models in the insurance sector, specifically in understanding policyholders’ acceptance of chatbots, provides insights into technology acceptance in service-oriented industries (Andrés-Sánchez & Gené-Albesa, 2023). Similarly, the integration of personality traits, like the Light Triad, into the traditional TAM framework indicates a growing interest in understanding the psychological factors influencing technology adoption behaviors (Leahy et al. This adaptation underscores the need to consider specific environmental and contextual factors influencing technology adoption in different settings.
Similarly, the exploration of technology acceptance in innovative environments like the metaverse for teaching anatomy demonstrates how these models can be applied to understand technology acceptance in emerging technological domains (Kalinkara & Özdemir, 2023). In the healthcare sector, the application of technology acceptance models to understand the adoption of e-learning technologies among clinical staff and telemedicine applications reflects the growing intersection of technology and healthcare (Stiller & Wager, 2023; Leelavathi, Manjunath, & Somasundaram, 2023). Additionally, the exploration of technology acceptance in consumer-focused industries, such as the beauty industry’s adoption of blockchain technology, provides an interesting perspective on technology acceptance outside the traditional domains of IT and healthcare (Kim & Jin, 2023). The exploration of the technology acceptance model in the context of customer engagement emphasizes the broader implications of technology acceptance on business and marketing strategies (Kumar, Banerjee, Al-Salti, & Upadhyaya, 2023). The healthcare sector continues to be a significant focus area for technology acceptance research, as evidenced by the studies on the adoption of e-learning technologies among clinical staff (Stiller & Wager, 2023) and the acceptance of telemedicine applications (Leelavathi, Manjunath, & Somasundaram, 2023).Moreover, the exploration of blockchain technology in the beauty industry by Kim and Jin (2023) reveals the expanding scope of technology acceptance beyond traditional IT and healthcare sectors.
This study illustrates how emerging technologies like blockchain can find relevance in consumer-focused industries, emphasizing the need for industry-specific acceptance models that cater to the unique characteristics and requirements of different sectors. The examination of the technology acceptance model in the realm of customer engagement by Kumar, Banerjee, Al-Salti, and Upadhyaya (2023) highlights the broader business implications of technology acceptance.
This perspective is essential for businesses and marketers as it underscores the importance of understanding how customer satisfaction and engagement are influenced by technology adoption and usage. This study tries to dive into the elements that influence the intention of FMCG firms to embrace such methods and contribute to sustainable business practices.
The Technology Acceptance Model (TAM), a pivotal framework in understanding technology adoption, presents a compelling rationale for studying the intention to adopt sustainability practices and technologies. Originating from Davis’s work on perceived usefulness and ease of use as primary determinants of technology acceptance, TAM offers a robust and adaptable tool for various technology-related studies, including sustainability technologies. This model’s relevance in the context of sustainability stems from its ability to dissect users’ perceptions, an essential aspect when considering the adoption of sustainable practices and technologies. TAM’s adaptability to different contexts, as evidenced in its application across various sectors, suggests its suitability for studying sustainability technologies. Integrating environmental concern into TAM, as proposed by some researchers, enhances the model’s applicability to sustainability. Studies on the acceptance of solar energy technologies, for instance, employ TAM to discern factors influencing individuals’ intentions to adopt these technologies, demonstrating the model’s effectiveness in predicting sustainable technology acceptance.
These additional factors consider social and psychological aspects influencing technology adoption, providing a comprehensive framework for understanding sustainable technologies and practices acceptance. In corporate settings, TAM’s application demonstrates its adaptability to different organizational cultures and values, crucial for promoting sustainability within corporate structures.
The significance of sustainable technology acceptance in healthcare, as indicated by TAM-based studies on telemedicine applications, highlights the potential of TAM in facilitating sustainable healthcare practices adoption. leveraging TAM to study the intention to adopt sustainability practices and technologies is well-founded. Its proven efficacy across sectors, ability to incorporate additional sustainability-related factors, and capacity to capture the nuances of technology acceptance in diverse contexts make it an ideal framework for understanding and encouraging the adoption of sustainable technologies and practices. Utilizing TAM, researchers, policymakers, and organizations can gain valuable insights into the factors influencing sustainability adoption, aiding the development of targeted strategies to foster a more sustainable and environmentally conscious future.
Literature Review
Fast-moving consumer goods companies are under growing pressure to implement sustainable practices across their value chains as a result of growing concerns. This chapter takes a look at the setting in which FMCG businesses intend to implement sustainability practices using the Technology Acceptance Model (TAM).
In the Fast-Moving Consumer Goods (FMCG) sector, environmental sustainability has become a paramount concern, driven by increasing consumer awareness and regulatory pressures. The application of the Technology Acceptance Model (TAM) in understanding and facilitating this transition towards sustainability is particularly relevant. The FMCG sector’s environmental impact is multi-dimensional, encompassing issues such as carbon emissions, water usage, waste generation, and the depletion of natural resources. Nyimbili and Chalwe (2023) highlight the importance of understanding the acceptance and adoption of new technologies and practices, which is crucial in the FMCG sector’s journey towards sustainability.
The adoption of eco-friendly technologies and sustainable business practices requires a deep understanding of the factors that drive organizational change and consumer behavior (Nyimbili & Chalwe, 2023). Andrés-Sánchez and Gené-Albesa (2023) underscore the importance of consumer acceptance of new technologies, such as biodegradable packaging and refillable containers, which align with the principles of TAM in terms of perceived usefulness and ease of use Davis (1989).
Similarly, the adoption of sustainable technologies in production processes, including energy-efficient machinery and water conservation systems, can be examined through the TAM lens (Andrés-Sánchez & Gené-Albesa, 2023). The complexity of these global supply chains requires a nuanced understanding of technology acceptance at various levels, from raw material sourcing to product distribution. Kemp (2023) suggests that an extended Educational Technology Acceptance Model (EETAM) could offer insights into how training and education in sustainability practices can be effectively implemented in corporate settings (Kemp, 2023) demonstrate the application of TAM in understanding workers’ acceptance of digital procedures, which can be extrapolated to understand employee acceptance of sustainable practices within the FMCG sector (Hendricks, Smith, Peres, & Sasangohar, 2023). Moreover, the FMCG sector is also exploring innovative solutions like blockchain technology for supply chain transparency and traceability.
Kim and Jin’s (2023) study on blockchain technology acceptance in the beauty industry illustrates how TAM can be applied to understand the acceptance of such innovative technologies in the FMCG sector (Kim & Jin, 2023). Çalışkan and Sevim (2023) show how the Technology Acceptance Model can be used to study the acceptance of service robots in hospitality, providing insights into how similar strategies could be employed in the FMCG sector to promote sustainable consumer practices (Çalışkan & Sevim, 2023). The FMCG sector’s transition towards environmental sustainability is a complex process that involves technological, behavioral, and strategic shifts.
The application of the Technology Acceptance Model (TAM) and its variants offers valuable insights into how these changes can be effectively implemented and accepted by various stakeholders, including consumers, employees, and supply chain partners. By understanding the factors that drive the acceptance of sustainable practices and technologies, the FMCG sector can better navigate the challenges and opportunities on the path to environmental sustainability.
Through the organization of innovative design practices, this set of activities guarantees the formalization of environmentally friendly themes. [Wagner, 2007] That is, the research has shown that proactive environmental strategies can give businesses a competitive edge, and activities at this level are seen as essential components of this strategy. Strategic environmental initiatives in businesses impact goals and metrics, which in turn increases staff sustainability consciousness, reducing wear and debris more effectively is one example of how to make processes more eco-friendly. For example, Nyimbili and Chalwe (2023) explore various models and theories related to technology acceptance and adoption, highlighting how external factors can be integrated into TAM to provide a more comprehensive understanding of technology acceptance (Nyimbili & Chalwe, 2023). Andrés-Sánchez and Gené-Albesa (2023) applied TAM to explain policyholders’ chatbot acceptance, demonstrating how user behavior towards new technology can be analyzed using the model. Their work illustrates how TAM can be adapted to specific technology contexts, offering insights into user acceptance and usage behavior (Andrés-Sánchez & Gené-Albesa, 2023). Furthermore, TAM has been applied in educational settings, as seen in the work of Kemp (2023), who designed an Extended Educational Technology Acceptance Model (EETAM).
This adaptation of TAM in an educational context highlights the model’s versatility and its applicability in different environments (Kemp, 2023). For instance, Zou and Huang (2023) examined the acceptance of ChatGPT among doctoral students, utilizing TAM to understand the factors influencing the acceptance of AI-based technologies in educational settings (Zou & Huang, 2023). Their research underscores the model’s relevance in understanding technology acceptance in healthcare, a sector increasingly reliant on digital solutions (Leelavathi, Manjunath, & Somasundaram, 2023). Moreover, TAM’s adaptability to different cultural and societal contexts is evident in studies like that of Abubakari, Zakaria, and Musa (2023), who explored digital learning acceptance in Islamic education. (2023) applied TAM to study workers’ acceptance of digital procedures, demonstrating the model’s utility in occupational settings (Hendricks, Smith, Peres, & Sasangohar, 2023). Furthermore, TAM has been extended to examine the psychological aspects of technology acceptance. (2023) explored the direct effects of the Light Triad on TAM, integrating personality traits into the model to provide a deeper understanding of user acceptance behavior (Leahy, Aplin-Houtz, Willey, Lane, Sharma, & Meriac, 2023). Kumar, Banerjee, Al-Salti, and Upadhyaya (2023) used TAM to explore the relationship between technology acceptance and customer engagement, highlighting the model’s relevance in a business context.
Comment on Literature Review
The Technology Acceptance Model (TAM) has two core components: Perceived Usefulness (PU) and Perceived Ease of Use (PEU). Over the years, researchers have extended TAM by adding additional constructs to better explain technology acceptance in specific contexts. Similarly, the Unified Theory of Acceptance and Use of Technology (UTAUT) model proposed by Venkatesh et al. (2003) integrated elements from several technology acceptance models, including TAM, and has been widely used in various studies. Several studies have applied TAM to understand the adoption of sustainable technologies. to explore consumer acceptance of electric vehicles, a critical component of environmental sustainability. In another study, focusing on renewable energy technologies they discovered that along with PU and PEU, factors such as environmental concern and government incentives played a significant role in technology acceptance.
To better understand the acceptance of sustainable technologies, researchers have extended TAM by including additional constructs for example, Bamberg (2013) proposed adding environmental concern as a factor influencing the perceived usefulness of sustainable technologies included social norms and attitude towards sustainability in their TAM-based model to study the adoption of green information technology. Depending on their operations and the items they sell to consumers, Fast Moving Consumer Goods (FMCG) firms can have a wide range of substantial effects when they implement environmental sustainability practices. Some important effects that fast-moving consumer goods firms would feel as a result of adopting environmental sustainability protocols are as follows: Businesses that priorities sustainability and provide environmentally friendly products can build a dedicated following of customers who share these values. Supply Chain Optimization, Waste Reduction, and Logistics Improvements are Common Components of Sustainable Business Practices. In 2022, Dwivedi and Paul published the concept of a circular economy (CE) is under increasing strain as people become more concerned about environmental sustainability. Enhanced efficiency and higher output are only two of the numerous advantages that accrue from implementing game-changing technology.
This is why digital supply chains (DSCs) are attractive to businesses operating in highly competitive global marketplaces. In order to maximize resource utilization and operational efficiencies, businesses constantly seek new technology advancements. Companies have been compelled to incorporate cutting-edge digital technology into their supply chains due to these situations. An assortment of technologies, including blockchain, AI, cloud computing, and the internet of things (IoT), have simplified the supply chain’s handling of large data, decision-making, feedback-gathering, opportunity-creating, etc. Innovative Products: Fast-moving consumer goods (FMCG) firms have the opportunity to create new products that are better for the environment. These products can include biodegradable packaging, sustainable materials, and formulas that use less energy. Undoubtedly, many management techniques are employed to enhance business performance, such as by means of more efficient allocation of resources.
Companies are increasingly using total quality management and other quality management practices to boost customer satisfaction and, by extension, business outcomes, especially in the operations management sector. Nowadays, many operational strategies incorporate environmental management. There is a lot of talk about how supply chain implementation impacts the environment, but research on how businesses may generate revenue to beat the competition is scant. Regulatory pressures in this area have been a major factor in the expansion of environmental management practices.
Sustainable practices can boost profit margins by reducing costs through energy efficiency, reducing waste, and optimizing resources. The increasing danger of carbon dioxide (CO2) emissions and the ensuing climate change has piqued the interest of developers in developing renewable and carbon-free energy sources in recent years. Despite the intermittent deployment of renewable energy in big enterprises, energy efficiency has increased in the industrial sector due to economic and environmental concerns.
Companies in the fast-moving consumer goods (FMCG) industry that place a premium on sustainability are in a stronger position to capitalize on the growing number of eco-conscious customers in emerging economies. In order to adopt sustainable practices, it is common to work with suppliers to guarantee the sustainability of components and raw materials.
A more sustainable supply chain and stronger supplier relationships can result from this. Reputation and customer trust may be enhanced by effectively communicating sustainability initiatives. Risk Mitigation: By implementing sustainable practices, organizations may lessen the likelihood of resource shortages, interruptions to their supply chains, and reputational harm caused by environmental events. Natural disasters, pandemics, process, supply, finance, information, and economic environment are some of the ways risks may be classified. Risk mitigation and resilience have been the primary areas of study at CSS in the past few years.
A risk mitigation portfolio must be developed and put into action in order to increase resilience as part of the company’s supply chain strategy. Researchers have looked at risk reduction from several angles, but the supply chain has been the primary emphasis. Companies may help achieve environmental goals by reducing their impact on the environment through the adoption of sustainable practices.
Understanding what motivates or deters individuals and organizations from adopting these technologies is crucial for designing effective interventions and policies to accelerate the transition towards a more sustainable future. Furthermore, the research problem extends to examining how external factors, such as environmental awareness, social norms, and policy incentives, interact with these core TAM constructs to influence technology acceptance.
Understanding the interplay of these external factors with individual perceptions is essential for developing a comprehensive view of sustainability technology adoption. In the context of this research, TAM serves as a foundational framework for exploring these various facets of technology acceptance in sustainability.
By applying and extending TAM, this research aims to provide valuable insights into the factors influencing the acceptance of sustainable technologies and practices, thereby addressing the overarching research problem. The research problem of this study revolves around understanding the multifaceted determinants of technology acceptance in the context of sustainability. It aims to uncover the psychological, social, and environmental factors that influence the adoption of sustainable technologies and practices, leveraging the robust framework of TAM.
The significance of this research lies in its potential to substantially contribute to our understanding of the factors influencing the adoption of sustainable technologies and practices, a critical concern in the face of global environmental challenges. By examining these factors through the lens of the Technology Acceptance Model (TAM), the study aims to provide insights that are pivotal for shaping effective policies, strategies, and interventions to encourage sustainable behavior in individuals and organizations. By understanding what drives or deters the adoption of sustainable technologies and practices, this study can inform the development of more effective strategies to mitigate the impacts of climate change, reduce carbon footprints, and conserve natural resources. Insights from this research can help in accelerating the adoption of renewable energy sources, sustainable transportation systems, and energy-efficient practices, all of which are essential for reducing greenhouse gas emissions and achieving environmental sustainability goals.
By providing insights into the factors that influence the acceptance of sustainable technologies, this research can inform the development of more effective policies and regulations that encourage the adoption of these technologies. This could include incentives for renewable energy adoption, regulations promoting energy-efficient practices, or initiatives to enhance public awareness and education about sustainability.
Research Questions:
Q1: To what extent does perceived usefulness influence FMCG enterprises’ intention to adopt environmental sustainability practices?
Q2: How does perceived ease of use impact FMCG enterprises’ intention to adopt environmental sustainability practices?
Conceptual Framework and hypothesis:
A conceptual model is suggested to shed light on the relationship between TAM constructions and the ambition to implement environmental sustainability practices in FMCG firms, expanding upon the current TAM framework. One factor that could sway workers’ decisions is perceived usefulness, which is based on how they see the advantages of sustainability. Just as how the perceived simplicity of incorporating sustainability into operations impacts intention, so does the perceived ease of usage. The theoretical framework establishes a connection between these concepts and lays forth a plan for future research into the FMCG industry’s sustainability adoption intention as it relates to TAM elements.
An extensive review of the relevant literature lays the groundwork for the next study in this chapter. The papers that were examined provide some background on TAM’s role in environmental sustainability and lay the groundwork for the conceptual framework that will be used to guide the empirical study in the chapters to follow.
Figure 2 diagrammatic representation of conceptual framework and hypothesis dimension.
Hypothesis 1:
- Null Hypothesis (H0): There is no significant relationship between perceived usefulness and the behavioral intention to adopt environmental sustainability practices in FMCG enterprises.
- Alternative Hypothesis (H1): There is a significant positive relationship between perceived usefulness and the behavioral intention to adopt environmental sustainability practices in FMCG enterprises.
Hypothesis 2:
- Null Hypothesis (H0): Perceived ease of use does not have a significant impact on the behavioral intention to adopt environmental sustainability practices in FMCG enterprises.
Alternative Hypothesis (H2): Perceived ease of use has a significant positive impact on the behavioral intention to adopt environmental sustainability practices in FMCG enterprises.
As shown in Figure 2, the conceptual framework postulates that the intention to adopt environmental sustainability practices is influenced by perceived utility and considered simplicity of use. The presented hypotheses are in line with this framework. (a graphical depiction of the hypothesis dimension and the conceptual framework). People are more inclined to plan to implement a behavior when they see it as beneficial, which is the primary premise of the TAM, as stated in Hypothesis 1. The second hypothesis elaborates on this by positing that people’s intentions are influenced by how easily sustainability practices may be integrated into current operations. The third hypothesis acknowledges the interaction between perceived utility and perceived ease of use, which together influence sustainability adoption intentions.
This study intends to investigate these hypotheses in order to find out how people’s views on the practicality and simplicity of environmental sustainability practices in FMCG companies affect their desire to use these practices. In the context of fast-moving consumer goods (FMCG) companies, this helps shed light on the elements that promote or discourage sustainable behavior.
Research Methodology
Addressing these concerns, this research applies the Technology Acceptance Model (TAM) as a theoretical lens to investigate FMCG firms’ intentions to adopt environmentally sustainable practices. Correlation analysis helps in understanding the relationship between the perceived usefulness and ease of use of sustainable practices and the intention to adopt them. Regression analysis further elucidates the strength and nature of these relationships, offering insights into how strongly each TAM component influences sustainability adoption intentions.
By understanding the factors that influence these companies’ acceptance of sustainable practices, this study provides a roadmap for FMCG firms to navigate the complexities of integrating environmental sustainability into their operations. By applying a modified version of the Technology Acceptance Model, the study sheds light on the factors that influence these companies’ intentions to adopt sustainable practices.
The exploration of the intricate relationship between the components of the Technology Acceptance Model (TAM) and the intention to adopt environmental sustainability practices within the Fast-Moving Consumer Goods (FMCG) industry is the focal point of this study. The deductive approach applied in this study involves testing the theoretical assertions of TAM in the specific context of environmental sustainability in the FMCG industry. The research then deduces hypotheses regarding how these TAM components might influence the intention to implement sustainability practices in the FMCG context. This research adopts a structured and methodical approach to investigate the role of TAM in shaping attitudes and intentions towards environmental sustainability in the FMCG sector. Through the use of deductive methodology, structured surveys, and statistical analysis, the study aims to provide valuable insights into how the components of TAM influence the desire to implement sustainable practices.
Questionnaire Design based on TAM Constructs:
In this comprehensive survey, designed to delve into the attitudes and intentions of professionals within the Fast-Moving Consumer Goods (FMCG) industry regarding environmental sustainability practices, respondents are invited to engage with a series of thoughtfully constructed questions. This survey aims to unravel the complex layers of perceived usefulness, perceived ease of use, and behavioral intention towards sustainable practices within the FMCG industry. Environmental Performance Enhancement: Here, the focus shifts to understanding if sustainable practices are perceived as key contributors to improving the company’s overall environmental performance. Environmental Impact Control and Management: The survey seeks to understand if adopting sustainability practices would lead to better control and management of environmental impacts. The survey then transitions to questions related to perceived ease of use, a crucial component in understanding how straightforward and feasible it is for FMCG companies to integrate sustainable practices into their operations:
Sample Size
This decision is informed by the guidelines provided by Saunders, Lewis, and Thornhill (2009), who suggest that a sample size of 66 is required to achieve a 95% confidence level with a 10% margin of error, given a target population of approximately 200. As Saunders, Lewis, & Thornhill (2009) note, the non-probability judgement sampling technique involves the purposive or deliberate selection of specific units from the universe to constitute a sample that represents the entire population. It allows for a more targeted approach to sampling, where the researcher’s judgement plays a crucial role in selecting cases that are deemed most representative or insightful for the research objectives.
The chosen sample size of 66, informed by Saunders, Lewis, and Thornhill’s guidelines, is optimized for robust statistical analysis within the confines of a manageable research process. Simultaneously, the adoption of non-probability judgement sampling allows for a focused and insightful exploration of environmental sustainability practices within the Egyptian FMCG industry, with the researcher’s expertise guiding the selection of the most pertinent and informative cases for study.
Measurements and Data Collection Process:
The study variables were measured through adapted measurement scales from previous studies as summarized in Table 1:
Table 1 Variables adapted measurement scales.
Construct | Dimension | Source |
Perceived Usefulness – Adoption of Environmental Sustainability |
1) Adopting environmental sustainability practices in our operations would lead to more efficient resource utilization. 2) The implementation of sustainable practices would improve the overall environmental performance of our company. 3) Integrating environmental sustainability into our business model would be beneficial for our long-term success. 4) Embracing environmental sustainability practices would enhance our company’s reputation and brand image. 5) Environmental sustainability adoption would contribute to cost savings and operational efficiency. 6) I believe that incorporating sustainable practices would positively impact the ecological footprint of our organization. 7) The adoption of environmental sustainability practices would provide us with greater control over our impact on the environment. 8) Adopting sustainability practices would be advantageous for our company’s alignment with eco-conscious consumers. 9) The utilization of environmental sustainability practices would allow us to better manage our environmental responsibilities. 10) I perceive that the adoption of environmental sustainability practices would be valuable for achieving our environmental goals.
|
Yang, Y., & Wang, X. (2019) and Alfadda, H. A., & Mahdi, H. S. (2021) |
Perceived Ease of Use – Adoption of Environmental Sustainability | 1) Learning to adopt environmental sustainability practices is straightforward for me.
2) Engaging in and transitioning to sustainable practices is quick and clear. 3) It’s simple to access resources and materials related to adopting environmental sustainability practices. 4) Overall, I find the process of adopting environmental sustainability practices to be user-friendly.
|
Yang, Y., & Wang, X. (2019) and Alfadda, H. A., & Mahdi, H. S. (2021) |
Behavioral Intention – Adoption of Environmental Sustainability | 1) I believe adopting environmental sustainability practices is beneficial for our organization.
2) Embracing environmental sustainability practices contributes to the improvement of our company’s ecological impact. 3) I feel confident in integrating environmental sustainability practices to enhance our business operations. 4) The materials and resources related to environmental sustainability practices are valuable for our organization. 5) I think adopting environmental sustainability practices should be a part of our future business strategies.
|
Yang, Y., & Wang, X. (2019) and Alfadda, H. A., & Mahdi, H. S. (2021) |
Elaborated by the researcher.
Moreover, the Interval scale represented in a five-point Likert scale which was ranged from 5= strongly agree to 1= strongly disagree has been utilized to know the degree of respondents’ agreement or disagreement with the proposed statements (Saunders, Lewis, & Thornhill, 2009).
The questionnaire will be administered electronically using an online survey platform. Participants will receive an invitation containing a link to the survey.
The data collection period is estimated to be four to six weeks, allowing sufficient time for participants to respond.
Data Collection and Analysis
The primary objective of this analysis is to unravel the facts and data, thereby yielding insightful findings that contribute to the understanding of environmental sustainability practices within the industry. The first step in the data analysis process involved ensuring the validity and reliability of the measuring scales used in the study. The use of this statistical test is crucial, as it ensures that the conclusions drawn from the study are based on reliable instruments. The results of the normality test inform the researcher about the appropriate statistical methods to use for further analysis. In the context of this study, multiple regression analysis was employed to investigate the relationships between the various components of the Technology Acceptance Model (TAM) – such as perceived usefulness, perceived ease of use, and behavioral intention – and the adoption of environmental sustainability practices in the FMCG industry. Starting from ensuring the validity and reliability of the measuring instruments to conducting descriptive and inferential statistical analyses, each step has been carried out with meticulous attention to detail.
Demographic analysis is a method for measuring racial, ethnic, and age distributions as well as how these characteristics have changed over time as a result of the three main demographic processes: migration, fertility, and mortality (Lucas, D. 2002).
Gender, years of experience in the FMCG business, and company size were the demographic factors measured in this research (Tables 3, 4, 5 & 5 and Figure 3). Gender was classified as either male or female; there were 66 men and 0 females. The respondents were divided into three groups based on their years of experience in the FMCG industry: 1-10, 10-20, and above 20. The frequency of each group was 23, 29, and 14 accordingly. The corresponding percentages are 34.8%, 43.9%, and 21.2%. There were three groups based on company size: small (4 occurrences), medium (11 occurrences), and big (51 occurrences). The corresponding percentages are 6.1%, 16.7%, and 77.3%.
Table 3 compared statistics. (Elaborated by the researcher based on SPSS results)
Table 4 Gender frequency table (Elaborated by the researcher based on SPSS results)
Table 5 Years of Experience frequency table (Elaborated by the researcher based on SPSS results)
Table 6 Company Size frequency table (Elaborated by the researcher based on SPSS results)
Figure 3 years of Experience and Company Size pie charters
Normality Test by Shapiro-Wilk
The Shapiro-Wilk test is a hypothesis test applied to a sample with the null hypothesis that the sample was generated from a normal distribution. If the p-value is low, we can reject this null hypothesis and say that the sample was not generated from a normal distribution.
Table 7 normality test (Elaborated by the researcher based on SPSS results)
Table 7 shows that all of the variables’ data substantially differ from a normal distribution, with p-values < 0.05 for every one of them. According to Razali and Wah (2011).
The distribution of the sample means approaches a normal distribution as the sample size rises, according to the Central Limit Theorem, even for a population with any distribution. Using a normal distribution as an approximation works pretty well for samples with an n-size greater than 30. With increasing n, the approximation approaches a normal distribution more closely. We have n = 66 here (Fischer, H. 2011).
A measuring system’s consistency, stability, and reliability may be assessed statistically using reliability analysis.
Table 8 CRONBACH’S ALPHA (Elaborated by the researcher based on SPSS results)
Overall | |||
Variables: | PU | PEOU | BI |
Table 8 reveals that the data have high levels of internal consistency dependability, with a total Cronbach’s alpha of 0.908. There is strong dependability and internal consistency among the 10 items on Perceived Usefulness, as indicated by Cronbach’s alpha score of 0.891. With a Cronbach’s alpha of 0.749, the four-question Perceived Ease of Use survey has a satisfactory level of internal consistency reliability. The Behavioral Intention scale has strong reliability and internal consistency with Cronbach’s alpha rating of 0.827.
Descriptive statistics is a subfield of statistics concerned with providing clear and concise summaries, organization, and presentation of data. Without drawing broad inferences about the population as a whole, the emphasis is on characterizing and analyzing the essential characteristics of a dataset. Table 9 measures the central tendency and variance, while Figure 4 shows the normal QQ plot of all variables.
Table 9 descriptive statistics (Elaborated by the researcher based on SPSS results)
A bivariate correlation study was performed on the variables listed in table 10. The Pearson’s correlation coefficient (r) was used to quantify the strength of the linear relationship between the two variables, which are quantitative and are paired. A correlation coefficient between 0.90 and 1.00 indicates a very high level of positive correlation, while a correlation between 0.70 and 0.90 indicates a high level of positive correlation, 0.50 to 0.70 indicates a moderate level of positive correlation, and a correlation between 0.30 and 0.50 indicates a low level of positive correlation.
there is a significant positive relationship between perceived usefulness (independent variable) and behavioral intention (dependent variable), the P value explored from the table is {P= .000 ˂ 0.01, r = 0.798}.
there is a significant positive relationship between perceived ease of use (independent variable) and behavioral intention (dependent variable), the P value explored from the table is {P= .000 ˂ 0.01, r = 0.464}.
Linear regression analysis has been conducted for each explanatory or independent variables with the response or dependent variable to analysis and predict the relationship between these two variables. It assumes a linear relationship between the independent variable and the dependent variable and aims to find the best fitting line that describes this relationship.
Linear regression shows a linear relationship between the independent (predictor) variable, which represents the x-axis, and the dependent (output) variable, which represents the y-axis.
To calculate the line of best fit, linear regression uses the traditional slope-intercept form the equation: (Yi = β0 + β1Xi) where Yi = dependent variable, β0 = constant/origin, β1 = slope/origin, Xi = independent variable.
Using data elaborated in the following tables 11, 12, 13. The results of a single regression model confirm that perceived usefulness has positive significant impact on behavioral intention (β = 0.798, P < 0.01). Additionally, it refers that a one unit increase in perceived usefulness will increase behavioral intention by 63.6 %. Moreover, (R2) is used to evaluate the model fit, generally, the model is considered fit when R2 equals to 0.10 or higher (Falk & Miller, 1992).
Giving the regression equation (Y = 0.678 + 0.817 X)
where, Y = behavioral intention and X = perceived usefulness. (Figures 5&6) confirm that values of the residuals have a distribution that is approximately normal.
Table 11 regression model summary H1
A single regression model’s results show that perceived ease of use significantly affects behavioural intention, according to the data shown in tables 14, 15, and 16. β = 0.464, P < 0.01. Furthermore, it states that a 21.5% increase in behavioural intention occurs for every one-unit rise in perceived usefulness. As an additional metric, (R2) is employed to assess the model’s fit; typically, a value of 0.10 or above indicates that the model is fit (Falk & Miller, 1992). With Y standing for behavioral intention and X for perceived ease of use, we get the regression equation (Y = 3.062 + 0.337 X).
Where, Y = behavioral intention and X = perceived ease of use the results show that the residuals follow a distribution that is close to normal (Figures 7 and 8).
Table 14 regression model summary H2
Both the perceived usefulness and the perceived ease of use, which are explanatory factors, and the response variable, behavioral intention, are the dependent variable in a multiple regression analysis. Tables 17, 18, and 19 provide the full results of the multiple regression model, which shows that perceived usefulness is the most important variable (63.6% of the total) and that perceived ease of use is the second most important (4.4%). Considering the total R2 value of perceived usefulness and perceived ease of use in model 2 reveals that both have a 68% impact on behavioral intention, indicating the optimal multiple regression model. Perceived usefulness and perceived simplicity of use significantly affect behavioral intention in a favorable way, according to this hypothesis. (P < 0.01), with β perceived usefulness = 0.723 and β perceived ease of use = 0.223.
Y = 0.425 + 0.741 X1 + 0.162 X2 is the regression equation that provides the relationship between behavioral intention, perceived usefulness (X1), and perceived ease of use (X2). The results show that the residuals follow a distribution that is close to normal (Figures 9&10).
Table 17 model summary of multiple regression
Table 18 multiple regression model ANOVA
Table 19 multiple regression model coefficients
The study, grounded in the Technology Acceptance Model (TAM), aims to unravel the intricacies of environmental sustainability practices within this sector. The subsequent multiple regression analysis, a staple in TAM research, was pivotal in understanding the relationships between the model’s components – perceived usefulness, perceived ease of use, and behavioral intention – and the adoption of environmental sustainability practices (Chen, Khan, Shiwakoti, Stasinopoulos, & Aghabayk, 2023). This chapter aims to discuss these findings in depth, linking them to existing literature and theories to provide a comprehensive understanding of the factors influencing environmental sustainability practices in the FMCG sector. The discussion will delve into how these findings align with or diverge from previous studies in similar contexts, such as the acceptance of digital technologies in education (Abubakari, Zakaria, & Musa, 2023) and the adoption of innovative practices in various industries (Ilyas, Uddin, Haleem, & Ahmad, 2023; Aplin-Houtz, Leahy, Willey, Lane, Sharma, & Meriac, 2023).
The insights garnered could inform strategies to enhance the adoption of sustainable practices in the industry, contributing to broader environmental and societal goals. This discussion is particularly relevant in the context of emerging trends and challenges in the FMCG sector, where understanding and addressing environmental concerns is becoming increasingly important (Kalinkara & Özdemir, 2023; Febriaryanti, 2023).
Correlation Analysis and Hypotheses Testing
The application of correlation analysis in this research is guided by the Technology Acceptance Model (TAM), which posits that perceived usefulness, perceived ease of use, and behavioral intention are key determinants in the adoption of new technologies or practices. The study’s hypotheses revolve around the relationships between perceived usefulness, perceived ease of use, and behavioral intention toward adopting sustainability practices. The first hypothesis (H1) postulates a significant positive relationship between perceived usefulness (independent variable) and behavioral intention (dependent variable). The correlation analysis, yielding a Pearson’s r of 0.798 and a p-value less than 0.01, strongly supports this hypothesis. This finding aligns with the existing literature, where perceived usefulness is consistently shown to be a strong predictor of behavioral intention (Nyimbili & Chalwe, 2023; Andrés-Sánchez & Gené-Albesa, 2023).
It suggests that in the Egyptian FMCG industry, the belief that sustainability practices are beneficial is likely to increase the intention to adopt these practices.The second hypothesis (H2) examines the relationship between perceived ease of use (independent variable) and behavioral intention (dependent variable). The correlation analysis reveals a lower, yet significant, positive correlation (Pearson’s r = 0.464, p-value < 0.01), indicating that ease of implementing sustainability practices also positively influences behavioral intention, albeit to a lesser extent than perceived usefulness.
Exploring Regression Analysis Results
The analysis confirmed a strong positive impact of perceived usefulness on behavioral intention. This suggests that in the FMCG industry, the more the sustainability practices are perceived as beneficial, the higher the likelihood of these practices being adopted. This finding aligns with existing literature that underscores the importance of perceived usefulness in technology acceptance and behavior change (Nyimbili & Chalwe, 2023; Andrés-Sánchez & Gené-Albesa, 2023). Moreover, the regression analysis indicated a significant, though comparatively weaker, positive effect of perceived ease of use on behavioral intention.
This implies that the ease with which sustainability practices can be implemented also plays a role in their adoption, albeit to a lesser degree than perceived usefulness. The regression coefficients obtained from the analysis offer a quantitative measure of the impact of each independent variable on the dependent variable. For example, a high regression coefficient for perceived usefulness indicates a strong positive influence on behavioral intention. Similarly, the model fit indices, such as R-squared, provide information on the proportion of variance in the dependent variable that is predictable from the independent variables. A high R-squared value would suggest that the model explains a large portion of the variance in behavioral intention, indicating a good fit.
Multiple Regression Analysis and Model Fit
The results of the multiple regression analysis revealed significant findings. Firstly, the analysis confirmed that both perceived usefulness and perceived ease of use have a significant impact on behavioral intention. This finding is consistent with the principles of TAM, which posits that these two factors are crucial determinants of technology acceptance and usage behavior (Nyimbili & Chalwe, 2023; Andrés-Sánchez & Gené-Albesa, 2023). The strength of these relationships, as quantified by the regression coefficients, provides valuable insights. A higher coefficient for perceived usefulness compared to perceived ease of use suggests that the former has a more substantial influence on the likelihood of adopting sustainability practices within the FMCG industry.
Theoretical Implications
The study’s findings enhance the theoretical understanding of TAM, particularly in the context of environmental sustainability. This alignment reaffirms TAM’s robustness and applicability in diverse contexts, extending beyond its traditional use in technology acceptance to encompass environmental sustainability practices in the FMCG industry. Additionally, the study enriches TAM by integrating it within the specific context of environmental sustainability.
Practical Implications
For FMCG companies, the findings provide crucial insights for designing strategies to promote environmental sustainability. Understanding that perceived usefulness is a strong predictor of behavioral intention implies that companies should focus on communicating the tangible benefits of sustainability practices. Additionally, the significance of perceived ease of use suggests that companies should aim to simplify the adoption of sustainability practices. Policymakers could develop incentives for companies that adopt sustainable practices or establish guidelines and standards that encourage the FMCG industry to move towards greater sustainability. Furthermore, the research findings offer valuable insights for marketing and communication strategies within FMCG companies. By understanding the factors that influence employees’ and consumers’ acceptance of sustainability practices, companies can tailor their communication strategies to address these factors effectively.
Comparative studies across various countries and regions could provide a broader understanding of the factors influencing environmental sustainability practices in the FMCG industry globally. Factors such as organizational culture, leadership styles, external environmental pressures, and consumer preferences could be examined to understand their impact on sustainability adoption in the FMCG industry. Further, exploring other theoretical frameworks or models that could explain sustainability practices in the FMCG industry would also be a valuable area of research.
Conclusions
More specifically, for fast-moving consumer goods (FMCG) companies, the perceived usefulness correlates positively with the behavioral intention to implement environmental sustainability practices (H1). Also, in FMCG companies, the behavioral intention to implement sustainable practices had a positive correlation with perceived ease of use (H2). When studying the implementation of environmental sustainability practices in the fast-moving consumer goods industry, it is crucial to consider how users perceive the practices’ usefulness and ease of use. By establishing the positive impact of perceived ease of use on the behavioral intention to adopt sustainability practices, the study advocates for the inclusion of user-friendly aspects in sustainability frameworks. The study contributes to a more holistic understanding of sustainability adoption by emphasizing the interplay between perceived usefulness, perceived ease of use, and behavioral intention. The findings of the study align with institutional theory, suggesting that the perceived benefits and ease of adopting sustainability practices contribute to the establishment of norms and expectations within the institutional environment.
The managerial implications of the research findings in the context of Egypt are multifaceted, offering practical guidance for FMCG enterprises operating within the Egyptian business environment. Implication: Managers should invest in strategic communication and training programs to enhance employees’ understanding of the benefits of environmental sustainability practices. Rationale: Clear communication and targeted training can increase the perceived usefulness of sustainability initiatives among employees, fostering a positive attitude and intention toward adoption. Implication: Managers should focus on implementing user-friendly strategies for sustainability practices, considering the local context and operational ease
- Abubakari, M., Zakaria, G., and Musa, J., 2023. Digital Learning Acceptance in Islamic Education: Validity and Reliability Testing of the Modified Technology Acceptance Model. Canadian Journal of Educational and Social Studies, 3, pp.27-42. DOI: 10.53103/cjess.v6i1.185.
- Alfadda, H.A. and Mahdi, H.S., 2021. Measuring students’ use of zoom application in language course based on the technology acceptance model (TAM). Journal of Psycholinguistic Research, 50(4), pp.883-900.
- Andrés-Sánchez, J. and Gené-Albesa, J., 2023. Explaining Policyholders’ Chatbot Acceptance with a Unified Technology Acceptance and Use of Technology-Based Model. Journal of Theoretical and Applied Electronic Commerce Research, 18, pp.1217-1227. DOI: 10.3390/jtaer18030062.
- Bagur‐Femenias, L., Llach, J. and del Mar Alonso‐Almeida, M., 2013. Is the adoption of environmental practices a strategical decision for small service companies? An empirical approach. Management Decision, 51(1), pp.41-62.
- Çalışkan, G. and Sevim, B., 2023. Use of service robots in hospitality: An observational study in terms of technology acceptance model. Tourism and Hospitality Research, pp.1-13. DOI: 10.1177/14673584231198438.
- Chen, Y. et al., 2023. Analysis of Australian Public Acceptance of Fully Automated Vehicles by Extending Technology Acceptance Model. Case Studies on Transport Policy, 14. DOI: 10.1016/j.cstp.2023.101072.
- Davis, F.D., 1989. Perceived usefulness, perceived ease of use, and user acceptance of information technology. MIS Quarterly, pp.319-340.
- Dwivedi, A. and Paul, S.K., 2022. A framework for digital supply chains in the era of circular economy: Implications on environmental sustainability. Business Strategy and the Environment, 31(4), pp.1249-1274.
- Falk, R.F. and Miller, N.B., 1992. A Primer for Soft Modeling. University of Akron Press.
- Fischer, H., 2011. A History of the Central Limit Theorem: From Classical to Modern Probability Theory (Vol. 4). New York: Springer.
- Hendricks, J. et al., 2023. Workers’ Acceptance of Digital Procedures: An Application of the Technology Acceptance Model. IISE Transactions on Occupational Ergonomics and Human Factors, 11, pp.1-21. DOI: 10.1080/24725838.2023.2240342.
- Ilyas, M. et al., 2023. Digital entrepreneurial acceptance: an examination of technology acceptance model and do-it-yourself behavior. Journal of Innovation and Entrepreneurship, 12. DOI: 10.1186/s13731-023-00268-1.
- Kalinkara, Y. and Özdemir, O., 2023. Anatomy in the metaverse: Exploring student technology acceptance through the UTAUT2 model. pp.1-18. DOI: 10.1002/ase.2353.
- Kemp, A., 2023. Design of an Extended Educational Technology Acceptance Model (EETAM). DOI: 10.13140/RG.2.2.21685.45285.
- Kim, N. and Jin, Y.-M., 2023. Effect of Beauty Industry’s Blockchain Technology Characteristics on Acceptance Intention Based on Technology Acceptance Model. Journal of the Korean Society of Cosmetology, 29, pp.912-924. DOI: 10.52660/JKSC.2023.29.4.912.
- Kumar, R. et al., 2023. Technology acceptance model and customer engagement: mediating role of customer satisfaction. Journal of Financial Services Marketing. DOI: 10.1057/s41264-023-00256-2.
- Leahy, S. et al., 2023. The Light Side of Technology Acceptance: The Direct Effects of the Light Triad on the Technology Acceptance Model. Journal of Managerial Issues, 35, pp.300-332
- Leelavathi, R., Manjunath, S., and Somasundaram, T., 2023. Customer Acceptance on Usage of Telemedicine Application: Technology Acceptance Model (TAM). DOI: 10.1007/978-3-031-43300-9_26.
- Lucas, D., 2002. The Scope of Demography. Asia-Pacific Population Journal, 5(3).
- Nyimbili, L. and Chalwe, M., 2023. A Review of Technology Acceptance and Adoption Models and Theories.
- Razali, N.M. and Wah, Y.B., 2011. Power comparisons of Shapiro-Wilk, Kolmogorov-Smirnov, Lilliefors and Anderson-Darling tests. Journal of Statistical Modeling and Analytics, 2(1), pp.21-33.
- Saunders, M., Lewis, P. and Thornhill, A., 2009. Research methods for business students. Pearson Education.
- Stiller, K. and Wager, S., 2023. Modelling e-learning acceptance of clinical staff using the General Extended Technology Acceptance Model for E-Learning. 11, pp.3084-3106.
- Venkatesh, V., Morris, M.G., Davis, G.B., and Davis, F.D., 2003. User acceptance of information technology: Toward a unified view. MIS Quarterly, pp.425-478.
- Wagner, M., 2007. Integration of environmental management with other managerial functions of the firm: empirical effects on drivers of economic performance. Long Range Planning, 40(6), pp.611-628.
- Yang, Y. and Wang, X., 2019. Modeling the intention to use machine translation for student translators: An extension of Technology Acceptance Model. Computers & Education, 133, pp.116-126.
- Zou, M. and Huang, L., 2023. Understanding doctoral students’ acceptance of ChatGPT in writing through technology acceptance model. Frontiers in Psychology, 14. DOI: 10.3389/fpsyg.2023.1259531.